ESG Strategist - Increasing climate performance without sacrificing bond returns
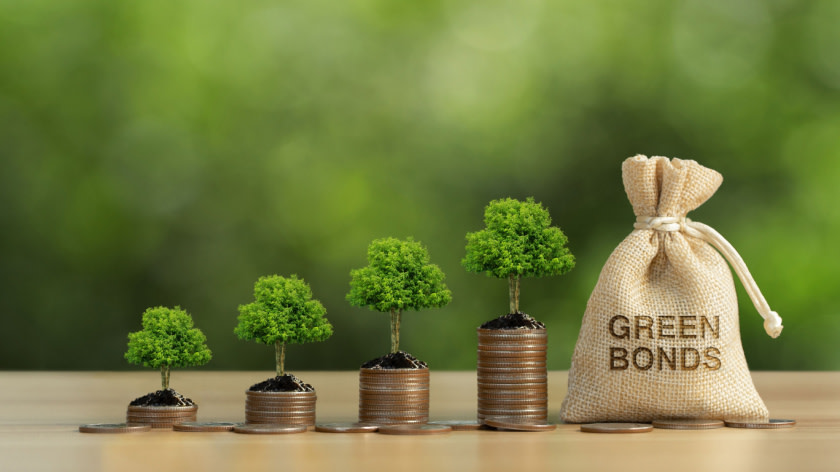
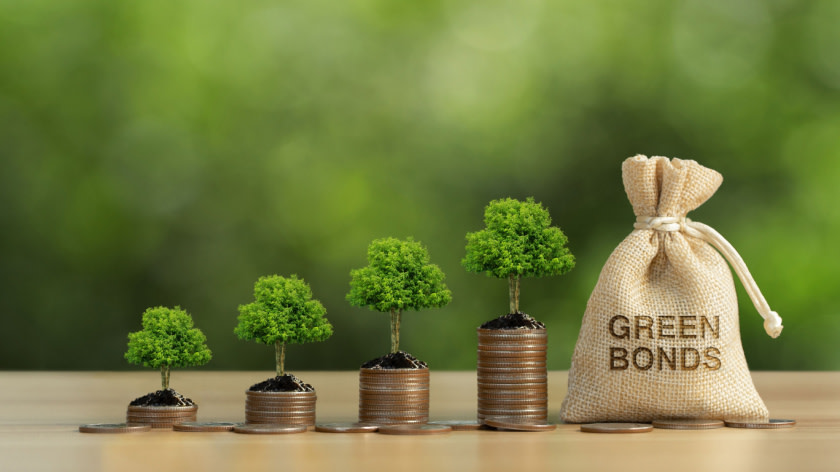
Investors often think that ESG investing comes at the expense of potential gains. However, by drawing upon portfolio optimization techniques, we demonstrate that it is possible to track a regular government bond benchmark’s performance while simultaneously generating positive climate impact. We also demonstrate that there seems to be a trade-off between the portfolio’s active risk and the climate performance. Hence, focusing on solely one ESG indicator may inadvertently compromise other ESG-related measures. The optimization process may lead to portfolios that heavily invest in illiquid bonds, prompting the need for additional financial constraints, which might require investors to potentially loosen some of the sustainability targets. Overall, our analysis aims to give investors some tools that explore how sustainability metrics can be considered alongside financial ones when optimizing a portfolio.
Fixed income investors often grapple with the perceived trade-off between financial returns and climate performance. Many in the financial community operate under the assumption that investing with a focus on environmental, social, and governance (ESG) criteria comes at the expense of potential gains. This view is underscored by, for example, the underperformance of several ESG-focused fixed income indices between 2020 and 2023. Such outcomes reinforce a common narrative: that investors must sacrifice returns to contribute positively to climate initiatives.
But is that really the case? In this piece, we try to challenge this notion. Drawing upon portfolio optimization techniques based on theoretical models, we explore whether it is possible to maintain existing fund performance (vis-à-vis a benchmark) while simultaneously enhancing positive climate impact. We note that the goal of this analysis is not to show a method for portfolio outperformance (that is – developing an ‘alpha’), but rather to highlight how index-tracking strategies can also deliver positive ESG impact. Investors do not need to sacrifice returns in order to achieve the latter. Lastly, our piece also explores whether such framework can be applied in practice, drawing on common market constraints, such as the fact that some bonds are significantly more liquid than others.
This piece builds on the framework developed by Kaul et. al (2021, see ). However, while Kaul et. al use a portfolio of only six sovereign issuers (Australia, Canada, Germany, Japan, UK, and the US), we aim to apply such framework to a more comprehensive sovereign bond portfolio, which is exclusively focused on the eurozone. In that sense, we demonstrate that such framework can also be applied to indices that are composed by a large number of sovereign issuers. Good to note that while we focus on sovereign bond portfolios, this framework can be replicated to any type of portfolio.
Methodology
Our portfolio optimization exercise builds on an existing sovereign benchmark, mainly the Bloomberg EuroAgg Treasury Index (Ticker: LEATTREU), which consists of Investment Grade bonds of 20 sovereign countries in the eurozone. We specify three climate-related tilts to this benchmark, which aims to create a new portfolio that will overweight bonds of countries that have ‘good’ ESG performance, and underweight bonds of countries that have ‘poor’ ESG performance (as per definition below). Ultimately, the climate-related indicators, as well as the number of such indicators included, should be at the portfolio manager’s discretion. The climate-related indicators - selected here only as an example - used to determine the tilting are:
The Germanwatch Climate Change Performance Index (CCPI, see here), which assesses a country’s performance against four categories: GHG emissions, energy, energy use and climate policy;
GHG emissions per capita (as of 2022, as per ISS ESG data); and
The ISS ESG’s Carbon risk rating (CRR), which rates sovereigns based on their efforts in implementing a climate policy framework, as well as country-wide GHG emission levels and their alignment with national and international reduction targets.
For the portfolio optimization, we use the Black and Litterman asset allocation model (1991, see for example here), which uses techniques that combine the market (i.e. the benchmark’s) returns with a specific investor view to derive the optimal asset allocation in a mean-variance optimization process. Mathematically speaking, this model aims to specify the ‘tilted’ portfolio’s weights by aiming to maximize the following:
The climate-related constraints could be summarized as following: we specify that the tilted portfolio should have a weighted CCPI score that is at least 10% higher than the benchmark, a weighted GHG emissions per capita that is at least 15% lower than the benchmark, and a combined carbon risk rating which is at least 20% higher than the benchmark. While these were the chosen climate-related indicators for this analysis, the list is not exhaustive. Investors are encouraged to consider additional indicators based on their specific objectives and preferences, allowing for flexibility in selecting the number and type of indicators that best align with their investment strategies.
Additional to the climate-related constraints, we also specify that (1) w_tilted≥5%*w_benchmark – that is, the weight of each country in the tilted portfolio should not be less than 5% than the current weight of that country in the benchmark (this ensures that no sovereign country has a weight of zero); and (2) that the duration of the tilted portfolio should be ±0.5 years from the duration of the benchmark portfolio (8.7y). Our optimization problem focuses on building a long-only portfolio (hence: the weights need to sum to 100%, and no short positions are allowed).
In other words, with this allocation model we are interested in determining the weights of each sovereign in a (tilted) portfolio, while ensuring that the risk-adjusted returns remain as close to the benchmark’s as possible and that some (financial and sustainability) constraints are satisfied.
For illustrative purposes only, our portfolio optimization is applied once – that is, over only a period of one month (June 2024). However, this strategy can easily be replicated for the upcoming periods with frequent rebalancing. We note that our analysis excludes any transaction costs, which are higher the more frequent the rebalancing chosen by the investor.
Results
As shown in the chart in the next page (left side), the optimization technique resulted in a portfolio overweight in countries that are more climate efficient according to the selected indicators (such as Greece, Portugal and the Netherlands), while underweighting countries that the indicators suggest have a ‘poor’ climate performance (such as France, Germany and Italy). This tilting in weights resulted in a portfolio with a weighted CCPI score and Carbon risk rating that is 10% and 20% higher than the benchmark, respectively, while also achieving a weighted GHG emissions per capita that is 15% lower than the benchmark.
As a next step, we evaluate the hypothetical performance of this portfolio. As we previously mentioned, our portfolio optimization is applied on a monthly basis and for illustrative purposes, we apply it once, mainly the month of June 2024. However, as we previously highlighted, this framework can be applied to any point in time: the goal is to get as close to the benchmark returns as possible, while achieving a certain pre-defined climate performance level.
Below we show the daily return (right), as well as the cumulative returns over this one month period (left chart). First take-away from the charts below is that the tilted portfolio tracks the performance of the benchmark very closely. In fact, at the end of this one-month period, we see that the tilted portfolio only underperformed the benchmark by 0.01% in terms of cumulative returns. The purpose of this exercise was not to find a alpha – that is, an approach that would make our tilted portfolio overperform the benchmark, but rather to investigate whether passive portfolio techniques (which include, for example, only a monthly rebalancing) could be sufficient to deliver the same amount of returns with a superior climate performance. A caveat to our analysis is that we do not consider transaction costs, which would reduce performance.
Another important aspect to this exercise is the risk-adjusted return. It is important that the tilted portfolio delivers similar returns without the investor having to undertake additional risk. That is what we explore below.
For that, we analyse two well-knowns measures: the Sharpe ratio, which evaluates the portfolio’s additional return for each unit of risk taken and how this compares to a risk-free investment; and the Information ratio (IR), which is similar to the Sharpe ratio, but measures the portfolio’s performance against a benchmark investment instead. The Sharpe ratio for the tilted portfolio is 0.005, which compares to 0.007 for the benchmark. A positive Sharpe ratio indicates that the portfolio’s return is higher than the risk-free rate when adjusted for the volatility of its returns, but the outperformance of the portfolios is almost negligible in this case. That is, as both Sharpe ratios are low, neither portfolio is providing significant excess returns on a risk-adjusted basis. The tilted portfolio has an IR of 0.01, which means that the portfolio has generated excess returns (in comparison to the benchmark) of 0.01 for every unit of risk. Overall, in line with our previous findings, the low IR indicates that the tilted portfolio does not significantly outperform the benchmark relative to the risk taken. This highlights once again that this technique of climate-friendly tilting a government bonds portfolio is appropriate for investors that aim to deliver similar (risk-adjusted) returns in comparison to a benchmark, but while providing a positive climate impact.
Furthermore, as this technique is usually backward-looking, we also evaluate the tilted portfolio’s performance one-month ahead. That is, using the optimal weighting that resulted from our optimization framework, we evaluate how the tilted portfolio performs relative to the benchmark. For the consequent month, the optimization would have to be re-applied.
This is demonstrated below. It is interesting to note that, while on a daily basis (chart on the right), the tilted portfolio continues to closely track the benchmark, it delivered a small outperformance on a cumulative basis. While we do not expect the climate tilting to result in an alpha per se (the framework aims to produce a portfolio that performs as close to the benchmark as possible – outperformance is not an input to the model), this exemplifies once again that investors should not necessarily sacrifice returns to achieve a superior climate performance.
Trade-offs: does higher risk mean higher climate performance?
As a next step, and once again building on the work from Kaul et. al (2021, see ), we want to investigate whether there is a trade-off between the portfolio’s climate performance and its active risk. The active risk (or tracking error) indicates the variability of a portfolio’s return relative to a benchmark. This is therefore based on the portfolio’s active returns, which are the returns of the tilted portfolio minus the benchmark. Under our current assessment, the active risk of the tilted portfolio is 0.03%, which indicates that returns of the tilted portfolio have a very low level of variability relative to the benchmark. However, we want to investigate whether a superior climate performance also results in the portfolio’s return becoming more volatile compared to the benchmark’s.
For that, we run the analysis aiming to maximize the climate performance, given certain risk restrictions. For example, we maximize CCPI subject to a certain level of active risk, while keeping all of our other non-climate constraints (e.g. related to duration) constant. The results are shown in the charts below.
The first observation we make from the chart above is that the higher the maximum CCPI for the tilted portfolio vis-à-vis the benchmark, given a set of non-climate constraints, the higher the active risk. However, the curvature of the graph indicates that while initial improvements of CCPI do not have a significant impact in the portfolio’s active risk, higher levels of improvement (especially beyond 15%) are associated with a sharp increase in active risk. At some point, there is a point of inflection in which there is only a marginal improvement of CCPI, but this comes at an expense of a marked increase in active risk.
Furthermore, we see that, as we increase CCPI and consequently, active risk, that this higher active risk also translates into a reduction in the portfolio’s weighted GHG emissions per capita, but only up until a certain level. That is, depending on the chosen active risk limit, maximizing CCPI for the portfolio could be detrimental to its per capita emissions. Interestingly, the portfolio’s per capita emissions becomes worse than the benchmark’s at a similar active risk level to where we see the inflection point of CCPI improvement per unit of additional active risk taken. This implies that, at some point, the tilting framework will overweight countries that have a slightly higher CCPI, but do not necessarily perform better in terms of GHG emission per capita (that is – the higher CCPI is not driven by lower emissions, but rather by other climate indicators of the country, such as climate policy).
A similar pattern is also seen when evaluating the trade-off between active risk and the portfolio’s carbon risk rating. Also here we see that at a certain active risk level, an additional unit of active risk taken results in a worsening of the portfolio’s carbon risk rating. Important to note however that, in contrast to the case with the GHG emissions per capita, the portfolio’s carbon risk rating remains higher than the benchmark’s at any level of active risk.
We also compare the trade-off between the portfolio’s active risk and its Sharpe ratio. As indicated in the chart in the previous page (right), the Sharpe ratio seems to increase as we undertake more risk. That is consistent with the fact that the more risk we take, the higher returns we achieve vis-à-vis the risk-free rate (although important to highlight that the increase in Sharpe ratio is almost negligible). Looking at IR, we see that at first, the more we increase active risk the more negative IR becomes, a trend which is slightly reversed as we move to a higher active risk level. Noteworthy is that in all scenarios, the IR remains negative, indicating that under a scenario where we aim to maximize CCPI, the portfolio’s risk-adjusted return is underperforming the benchmark’s.
Overall, the analysis above highlights the importance of considering a range of climate indicators when evaluating the climate performance of a portfolio. Focusing solely on maximizing one metric (such as in our case the CCPI) may inadvertently compromise other ESG-related measures. Investors should examine levels at which all indicators reflect a superior climate performance in comparison to the benchmark, while also not necessarily resulting in underperformance against the benchmark. That is why the optimization model presented earlier provides investors with a good illustration of a holistic framework that incorporates different factors simultaneously, resulting in an enhanced climate performance portfolio that does not sacrifices overall returns (excluding transaction costs).
Application of the tilting method in practice
One question that arises from this exercise is: could it be applied in practice? For example, one of the limitations of the above analysis is that is does not include transaction costs that arise from a frequent rebalancing. Furthermore, as we have shown in the previous page, the tilted portfolio is overweight countries such as Greece, Portugal and the Netherlands, and underweight countries such as France, Germany and Italy. Hence, it might be difficult for a portfolio manager to replicate such strategy due to liquidity constraints (for example: German Bunds and French OATs are significantly more liquid than Portuguese PGBs and the Greek GGBs). As the size of these markets are smaller, investors might face difficulties while trying to purchase a large amount of these bonds.
With that in mind, we re-ran the optimization framework aiming to add additional weight restrictions to a more liquid country, such as Germany. We first replicate the analysis by adding a restriction that the combined weight within German Bunds should not be below 50% of the weight it currently receives in the benchmark. Mathematically, this is stated as the following: w_(tilted DE)≥50%*w_(benchmark DE). This ensures that we at least keep a significant portion of our portfolio invested in a liquid asset, such as German government bonds. As an additional scenario, we also re-ran the analysis by assuming that the investment in any country should not exceed 40%. In this case, the following constraint should be satisfied: 5%*w_benchmark ≤ w_tilted≤40%.
Unfortunately, under these restrictions, it was only possible to find a solution that satisfies all the constraints if we are willing to loosen the sustainability targets of the tilted portfolio. The resulting weights under each of these scenarios are presented below.
Under the first scenario (which assumes w_(tilted DE)≥50%*w_(benchmark DE)) the resulting tilted portfolio has a CCPI that is 11% higher than the benchmark’s, but GHG emissions per capita that are only 9% lower and a CCR that is 11% better. Hence, the two latter sustainability criteria had to be loosened in order for the new restriction to be met (our initial exercise required the tilted portfolio to have GHG emissions per capita that are only 15% lower and a CCR that is 20% better). With regards to our second scenario, the resulting portfolio has very similar sustainability metrics (as it can be observed from the graphs above, the tilted weights are also very close to each other). However, as the weight towards Germany is less restrictive, the portfolio achieves a slightly better climate performance, with GHG emissions per capita that are only 10% lower than the benchmark portfolio and a CCR that is 13% better.
Looking at the performance of these tilted portfolios over the month of June, the trend we have seen previously is repeated. While the tilted portfolios slightly underperform at the start of the month, they overperform thereafter, consequently resulting in a cumulative return that is very similar to the benchmark (-0.23% vs. -0.20%).
What about additional liquidity constraints?
The aforementioned analysis applies a constraint to the weight allocated to German Bunds as a way to tackle the liquidity issue of this framework. However, by applying a restriction to only one sovereign, the tilted portfolio is still very overweight (illiquid) countries such as Greece and the Netherlands, as it is possible to see from the charts above. Therefore, one could say that the tilted portfolio still faces significant liquidity issues. Hence, we also investigate whether it is possible to run the optimization framework by adding also a restriction to two other more liquid sovereign bond issuers: Italy and France.
Similar to before, we therefore consider the following constraints:
Once again, under these restrictions, it was only possible to find a solution that satisfies all the constraints if we are willing to loosen (even more) the sustainability targets of the tilted portfolio. The resulting weights under each of these scenarios are presented below.
In this case, the resulting portfolio has a combined 32% invested in German Bunds, French OATs and Italian BTPs. Furthermore, no country receives a weight that exceeds 40%, satisfying also therefore our previous second constraint (5%*w_benchmark ≤ w_tilted≤40%). In terms of climate performance, the tilted portfolio has a CCPI that is 8% better than the benchmark, GHG emissions per capita that are 5% lower and CCR that is 12% better. Interestingly, the more we add a weight restriction to these countries, the lower the sustainability performance of the portfolio (see chart above on the right). That is because the liquid countries – Germany, Italy and France – do not perform well in some of the climate indicators we chosen. For example, Italy has a significantly lower CCPI than the average and median across all countries (50.6 vs 59.0 of median and 59.8 of average), while Germany has a significantly higher GHG emission per capita (10.5 tons/per capita, vs 8.6 of median and 9.2 of average).
In terms of returns, as the optimization framework aims to maximize returns so that those are as close as possible to the benchmark’s performance, the resulting tilted portfolio performance (in all aforementioned constraints) is very similar to the benchmark’s.
Conclusion
In conclusion, our analysis demonstrates that it is feasible to enhance the climate performance of a sovereign bond portfolio without significantly compromising financial returns. By leveraging on portfolio optimization techniques, particularly the Black and Litterman model, investors can build portfolios that closely mirror the performance of a standard benchmark, while simultaneously achieving enhanced climate outcomes and meeting certain financial constraints.
Our study also sheds light on the trade-offs between improving climate performance and managing portfolio risk. While initial improvements in climate metrics do not significantly increase active risk beyond a certain threshold, further enhancements lead to a sharp rise in risk, suggesting diminishing returns on climate metrics relative to risk. This finding underscores the importance of a balanced approach that considers a broad range of ESG indicators, rather than focusing on a single metric, to avoid unintended negative impacts on other aspects of the portfolio’s climate performance.
Furthermore, we show that the application of such framework does not come without its challenges. For example, the optimization process might result in a portfolio that heavily invests in illiquid bonds. In this case, investors might want to consider imposing additional financial constraints that address this liquidity issue. However, doing so could inadvertently impact the tilted portfolio’s climate performance.
Lastly, important to note that the portfolio’s climate tilting is closely tied to the investor’s selection of which climate indicators to apply in the optimization framework. For example, our choice to add indicators that emphasize carbon emissions naturally results in a portfolio that overweights bonds from peripheral countries and underweights bonds from core countries. Investors who are more concerned about exposure to physical risk or the fiscal impact from different investment needs for the energy transition might want to consider adding other indicators to reflect these aspects. Nevertheless, the advantage of using such a framework is that investors do not need to solely rely on one climate indicator. In a previous piece (see ) we highlighted how ESG scores for sovereigns tend to overweight the ‘G’ pillar. Hence, by using portfolio optimization techniques, investors can rely on a wide set of climate-related metrics, chosen as per the portfolio manager’s discretion, ultimately reducing the dependence on such ‘G’ pillar scores that may not draw a complete picture of a country’s climate performance.
Nevertheless, even under a scenario where investors need to relax certain sustainability targets in order to achieve a portfolio that better suits their preferences, the overall framework applied in this piece could provide a valuable tool for investors. It allows for investors to align their portfolios with both financial and sustainability goals, ultimately enabling them to contribute to a more climate-resilient investment strategy without sacrificing returns.